Evaluación de Flecha Seca en palma aceitera (Elaeis guineensis Jacq.) mediante imágenes multiespectrales, Costa Rica
DOI:
https://doi.org/10.15517/am.v33i2.47557Palabras clave:
teledetección, índices de vegetación, índice simple ratioResumen
Introducción. El uso de la teledetección para identificar los diferentes estados de la salud de los cultivos, así como su relación con el rendimiento, constituye una herramienta muy importante en la implementación de la Agricultura de Precisión. Objetivo. Relacionar el estado fitosanitario, obtenido por expertos mediante la evaluación visual, de plantas de palma de aceite (Elaeis guineensis Jacq.) afectadas por el síndrome de Flecha Seca (SFS), con los índices de vegetación calculados a partir de imágenes multiespectrales obtenidas con un vehículo aéreo no tripulado (VANT). Materiales y métodos. El estudio se desarrolló en una plantación de cuatro hectáreas con palma de aceite de tres años de trasplantada, propiedad de CoopeCalifornia R.L. ubicada en Parrita, Costa Rica. Se realizaron cuatro evaluaciones visuales del estado del SFS de diciembre de 2014 a febrero de 2017. Con estas evaluaciones se obtuvo la evolución espacio-temporal de la incidencia de la SFS durante veintiséis meses. En la última evaluación se realizó un vuelo con un VANT que portaba una cámara multiespectral Parrot Sequoia, con la cual se calcularon los índices de vegetación que luego fueron relacionados con el estado de SFS de las plantas. Resultados. Se encontró una alta variabilidad espacial y temporal del SFS durante todas las evaluaciones visuales. También se logró encontrar una fuerte relación entre los datos de las evaluaciones obtenidas en campo con los datos generados a partir de sensores remotos. El índice de vegetación Simple Ratio (SR) mostró diferencias significativas entre las plantas clasificadas como sanas y las plantas clasificadas con SFS, con grados 2 y 3 de severidad. Conclusiones. Es posible relacionar datos de campo, obtenidos mediante criterio experto, con información multiespectral de alta resolución espacial para identificar el SFS en plantaciones comerciales de palma aceitera.
Descargas
Citas
Acosta, A., & Munévar, F. (2003). Bud rot in oil palm plantations: link to soil physical properties and nutrient status. Better Crops International, 17(2), 22–25.
Arnal Barbedo, J. G. (2019). A review on the use of unmanned aerial vehicles and imaging sensors for monitoring and assessing plant stresses. Drones, 3(2), Article 40. https://doi.org/10.3390/drones3020040
Birth, G. S., & McVey, G. R. (1968). Measuring the color of growing turf with a reflectance spectrophotometer. Agronomy Journal, 60(6), 640–643. https://doi.org/10.2134/agronj1968.00021962006000060016x
Calera, A., Campos, I., Osann, A., D’Urso, G., & Menenti, M. (2017). Remote sensing for crop water management: From ET modelling to services for the end users. Sensors, 17(5), Article 1104. https://doi.org/10.3390/s17051104
Campbell, J. B., & Wynne, R. H. (2011). Introduction to remote sensing (5th Ed.). Guilford Press.
Ceccato, P., Flasse, S., Tarantola, S., Jacquemoud, S., & Grégoire, J. M. (2001). Detecting vegetation leaf water content using reflectance in the optical domain. Remote Sensing of Environment, 77(1), 22–33. https://doi.org/10.1016/S0034-4257(01)00191-2
Chinchilla, C. M. (2008). Las muchas caras de las pudriciones del cogollo (y de flechas) en palma aceitera y la importancia de un enfoque integral para su manejo. ASD Oil Palm Papers, 32, 11–23. https://bit.ly/3IaSUCx
Chuvieco, E. (2008). Teledetección ambiental (3a ed.). Ariel Ciencia.
Environmental Systems Research Institute. (2021). Cómo funciona Autocorrelación espacial (I de Moran global). ArcGIS. https://bit.ly/350f63Z
Exelis Visual Information Solutions, Inc. (2016). Broadband Greenness. L3HARRIS. https://bit.ly/3LP8czl
Fernández-Arango, D., Martín-Isabel, P., Vilar del Hoyo, L., & Pacheco-Labrador, J. (2015). Estimación del contenido de humedad de la vegetación a partir de imágenes hiperespectrales adquiridas por el sensor aeroportado CASI (Compact Airborne Spectrographic Imager). Geofocus: Revista Internacional de Ciencia y Tecnología de la Información Geográfica, 16, 177–204. http://www.geofocus.org/index.php/geofocus/article/view/399
Ferguson, R., & Rundquist, D. (2018). Remote sensing for site-specific crop management. In D. K. Shannon, D. E. Clay, & N. R. Kitchen (Eds.), Precision agriculture basics (pp. 103–117). American Society of Agronomy, Crop Science Society of America, & Soil Science Society of America. https://doi.org/10.2134/precisionagbasics.2016.0092
Gitelson, A. A., & Merzlyak, M. N. (1998). Remote sensing of chlorophyll concentration in higher plant leaves. International Journal of Remote Sensing, 18(12), 2691–2697. https://doi.org/10.1080/014311697217558
Gitelson, A. A., Merzlyak, M. N., & Chivkunva, O. B. (2001). Optical properties and nondestructive estimation of anthocyannin content in plant leaves. Photochemistry and Photobiology, 74(1), 38–45. https://doi.org/10.1562/0031-8655(2001)074<0038:opaneo>2.0.co;2
Gogoi, N. K., Deka, B., & Bora, L. C. (2018). Remote sensing and its use in detection and monitoring plant diseases: A review. Agricultural Reviews, 39(4), 307–3013. https://doi.org/10.18805/ag.r-1835
Gröll, K., Graeff, S., & Claupein, W. (2007, März 5-7). Use of vegetation indices to detect plant diseases [Conference presentation Referate der 27]. Agrarinformatik im Spannungsfeld zwischen Regionalisierung und globalen Wertschöpfungsketten, Stuttgart, Germany. https://bit.ly/33JE72Y
Hennessy, A., Clarke, K., & Lewis, M. (2020). Hyperspectral classification of plants: A review of waveband selection generalisability. Remote Sensing, 12(1), Article 113. https://doi.org/10.3390/rs12010113
Huete, A. R. (1988). A Soil-Adjusted Vegetation Index (SAVI). Remote Sensing of Environment, 25, 295–309. https://doi.org/10.1016/0034-4257(88)90106-X
Instituto Meteorologico Nacional del Costa Rica. (2021). Datos climaticos estación de Damas en Aguirre. https://www.imn.ac.cr/mapa
Kaufman, Y. J., & Tanre. D. (1992). Atmospherically Resistant Vegetation Index (ARVI) for EOS-MODIS. IEEE Transactions on Geoscience and Remote Sensing, 30(2), 261–270. https://doi.org/10.1109/36.134076
Khanal, S., Kushal, K. C., Fulton, J. P., Shearer, S., & Ozkan, E. (2020). Remote sensing in agriculture—accomplishments, limitations, and opportunities. Remote Sensing, 12(22), Article 3783. https://doi.org/10.3390/rs12223783
Liaghat, S., Ehsani, R., Mansor, S., Shafri, H. Z. M., Meon, S., Sankaran, S., & Azam, S. H. M. N. (2014). Early detection of basal stem rot disease (Ganoderma) in oil palms based on hyperspectral reflectance data using pattern recognition algorithms. International Journal of Remote Sensing, 35(10), 3427–3439. https://doi.org/10.1080/01431161.2014.903353
Lillesand, T. M., Kiefer, R. & Chipman, J. (1987). Remote sensing and image interpretation (2nd ed.). John Wiley & Sons.
Loong Chong, K., Devi Kanniah, K., Pohl, C., & Pang Tan, K. (2017). A review of remote sensing applications for oil palm studies. Geo-Spatial Information Science, 20(2), 184–200. https://doi.org/10.1080/10095020.2017.1337317
Mahlein, A. K. (2016). Present and future trends in plant disease detection. Plant Disease, 100(2), 241–251. https://doi.org/10.1094/PDIS-03-15-0340-FE
Mahlein, A. K., Kuska, M. T., Behmann, J., Polder, G., & Walter, A. (2018). Hyperspectral sensors and imaging technologies in phytopathology: state of the art. Annual Review of Phytopathology, 56, 535-558. https://doi.org/10.1146/annurev-phyto-080417-050100
Mahlein, A. K., Rumpf, T., Welke, P., Dehne, H. W., Plümer, L., Steiner, U., & Oerke, E. C. (2013). Development of spectral indices for detecting and identifying plant diseases. Remote Sensing of Environment, 128, 21–30. https://doi.org/10.1016/j.rse.2012.09.019
Martínez-Barbáchano, R., & Solís-Miranda, G. A. (2018). Caracterización espectral y detección de Flecha Seca en palma africana en Puntarenas, Costa Rica. Revista Geográfica de América Central, 61(2), 349-377. https://doi.org/10.15359/rgac.61-2.13
Mata, R., Rosales, A., Sandoval, D., Vindas, E., & Alemán, B. (2020). Subórdenes de suelos de Costa Rica [mapa digital, escala 1:200000]. Universidad de Costa Rica. http://www.cia.ucr.ac.cr/?page_id=139
Petropoulos, G., & Kalaitzidis, C. (2012). Multispectral vegetation index in remote sensing: An overview. In W. Zhang (Ed.), Ecological modeling (pp. 15–34). Nova Science Publishers, Inc.
Pinter, P. J., Hatfield, J. L., Schepers, J. S., Barnes, E. M., Moran, M. S., Daughtry, C. S., & Upchurch, D. R. (2003). Remote sensing for site-specific crop management. Photogrammetric Engineering & Remote Sensing, 69(6), 647–664.
Pix4D SA. (2021). Pix4DMapper®. https://www.pix4d.com/es/acerca-de-pix4d
Roujean, J. L., & Breon, F. M. (1995). Estimating PAR absorbed by vegetation from bidirectional reflectance measurements. Remote Sensing of Environment, 51(3), 375–384. https://doi.org/10.1016/0034-4257(94)00114-3
Rouse, J. W., Haas, R. H., Schell, J. A., & Deering, D. W. (1974). Monitoring vegetation systems in the great plains with ERTS (Paper A 20). In S. C. Freden, E. P. Mercanti, & M. A. Becker (Eds.), Third Earth Resources Technology Satellite-1 Symposium. Volume 1: Technical Presentations, section A (pp. 301–317). NASA. https://ntrs.nasa.gov/citations/19740022614
Sachs, J. D., Remans, R., Smukler, S. M., Winowiecki, L., Andelman, S. J., Cassman, K. G., Castle, D., DeFries, R., Denning, G., Fanzo, J., Jackson, L. E., Leemans, R., Lehmann, J., Milder, J. C., Naeem, S., Nziguheba, G., Palm, C. A., Pingali, P. L., Reganold, J. P., … Sanchez, P. A. (2012). Effective monitoring of agriculture: A response. Journal of Environmental Monitoring, 14(3), 738–742. https://doi.org/10.1039/c2em10584e
Santoso, H., Gunawan, T., Jatmiko, R. H., Darmosarkoro, W., & Minasny, B. (2011). Mapping and identifying basal stem rot disease in oil palms in North Sumatra with QuickBird imagery. Precision Agriculture, 12(2), 233–248. https://doi.org/10.1007/s11119-010-9172-7
Santoso, H., Tani, H., & Wang, X. (2016). A simple method for detection and counting of oil palm trees using high-resolution multispectral satellite imagery. International Journal of Remote Sensing, 37(21), 5122–5134. https://doi.org/10.1080/01431161.2016.1226527
Shafri, H. Z. M., & Hamdan, N. (2009). Hyperspectral imagery for mapping disease infection in oil palm plantation using vegetation indices and red edge techniques. American Journal of Applied Sciences, 6(6), 1031–1035. https://doi.org/10.3844/ajassp.2009.1031.1035
Sishodia, R. P., Ray, R. L., & Singh, S. K. (2020). Applications of remote sensing in precision agriculture: A review. Remote Sensing, 12(19), Article 3136. https://doi.org/10.3390/rs12193136
Sripada, R. P., Heiniger, R. W., White, J. G., & Meijer, A. D. (2006). Aerial color infrared photography for determining early in-season nitrogen requirements in corn. Agronomy Journal, 98(4), 968–977. https://doi.org/10.2134/agronj2005.0200
Tao, H., Feng, H., Xu, L., Miao, M., Long, H., Yue, J., Li, Z., Yang, G., Yang, X., & Fan, L. (2020). Estimation of crop growth parameters using UAV- based hyperspectral remote sensing data. Sensors, 20(5), Article 1296. https://doi.org/10.3390/s20051296
Thenkabail, P., Lyon, J., & Huete, A. (2011). Advances in hyperspectral remote sensing of vegetation and agricultural croplands. In P. Thenkabail, J. Lyon, & A. Huete (Eds.), Hyperspectral remote sensing of vegetation (pp. 3–36). CRC Press.
Ustin, S. L., Roberts, D. A., Gamon, J. A., Asner, G. P., & Green, R. O. (2004). Using imaging spectroscopy to study ecosystem processes and properties. BioScience, 54(6), 523–534. https://doi.org/10.1641/0006-3568(2004)054[0523:UISTSE]2.0.CO;2
Van de Lande, H. L., & Zadoks, J. C. (1999). Spatial patterns of spear rot in oil palm plantations in Surinam. Plant Pathology, 48(2), 189–201. https://doi.org/10.1046/j.1365-3059.1999.00331.x
Viera-Torres, M., Sinde-González, I., Gil-Docampo, M., Bravo-Yandún, V., & Toulkeridis, T. (2020). Generating the baseline in the early detection of bud rot and red ring disease in oil palms by geospatial technologies. Remote Sensing, 12(19), Article 3229. https://doi.org/10.3390/rs12193229
Weiss, M., Jacob, F., & Duveiller, G. (2020). Remote sensing for agricultural applications: A meta-review. Remote Sensing of Environment, 236, Article 111402. https://doi.org/10.1016/j.rse.2019.111402
Zhang, J., Huang, Y., Pu, R., Gonzalez-Moreno, P., Yuan, L., Wu, K., & Huang, W. (2019). Monitoring plant diseases and pests through remote sensing technology: A review. Computers and Electronics in Agriculture, 165, Article 104943. https://doi.org/10.1016/j.compag.2019.104943
Zhao, H., Yang, C., Guo, W., Zhang, L., & Zhang, D. (2020). Automatic estimation of crop disease severity levels based on vegetation index normalization. Remote Sensing, 12(12), Article 1930. https://doi.org/10.3390/rs12121930
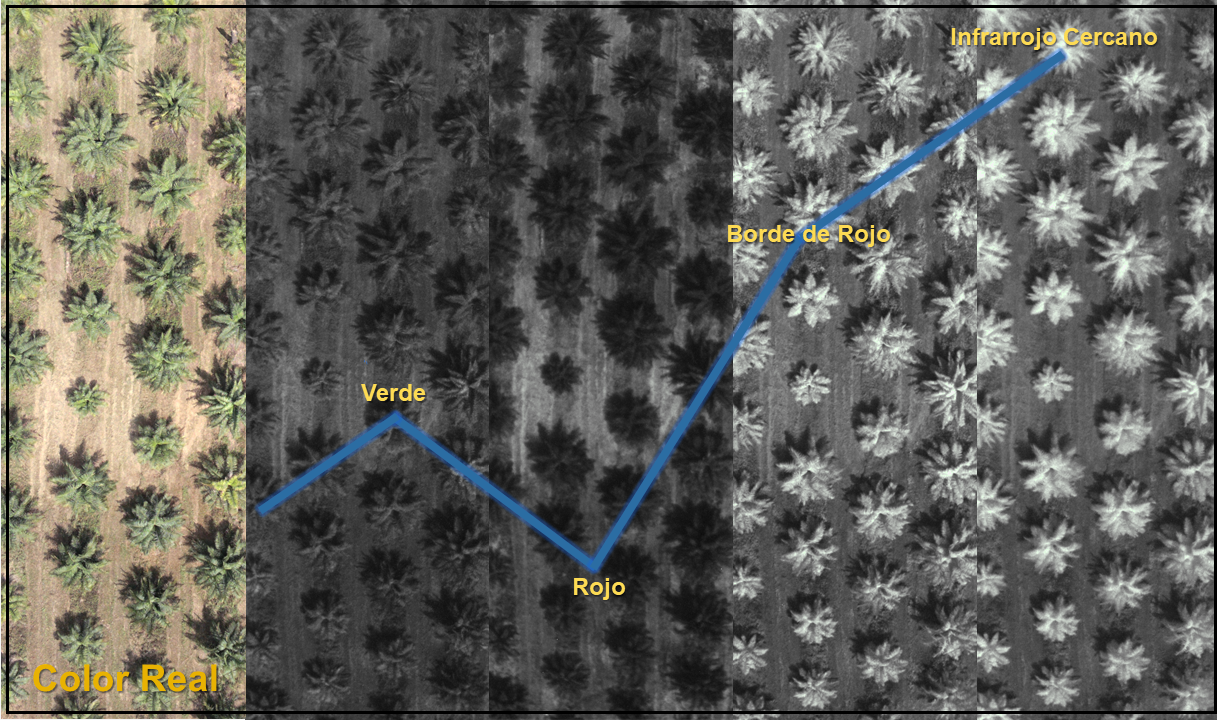
Archivos adicionales
Publicado
Cómo citar
Número
Sección
Licencia
1. Política propuesta para revistas de acceso abierto
Los autores/as que publiquen en esta revista aceptan las siguientes condiciones:
- Los autores/as conservan los derechos morales de autor y ceden a la revista el derecho de la primera publicación, con el trabajo registrado con la licencia de atribución, no comercial y sin obra derivada de Creative Commons, que permite a terceros utilizar lo publicado siempre que mencionen la autoría del trabajo y a la primera publicación en esta revista, no se puede hacer uso de la obra con propósitos comerciales y no se puede utilizar las publicaciones para remezclar, transformar o crear otra obra.
- Los autores/as pueden realizar otros acuerdos contractuales independientes y adicionales para la distribución no exclusiva de la versión del artículo publicado en esta revista (p. ej., incluirlo en un repositorio institucional o publicarlo en un libro) siempre que indiquen claramente que el trabajo se publicó por primera vez en esta revista.
- Se permite y recomienda a los autores/as a publicar su trabajo en Internet (por ejemplo en páginas institucionales o personales) antes y durante el proceso de revisión y publicación, ya que puede conducir a intercambios productivos y a una mayor y más rápida difusión del trabajo publicado (vea The Effect of Open Access).