Estimación de la humedad del suelo mediante regresiones lineales múltiples en Llano Brenes, Costa Rica
DOI:
https://doi.org/10.15517/am.v33i2.47872Palabras clave:
contenido de agua en el suelo, Coffea, zona tropicalResumen
Introducción. La humedad del suelo es una variable muy importante en el suministro de agua para la agricultura y es su principal recurso. Sin embargo, su medición en campo suele presentar limitaciones, por lo que su predicción es necesaria para diversas actividades de planificación agrícola e investigación. Objetivo. Predecir la humedad diaria del suelo a escala de cultivo, a partir de información meteorológica mediante modelos de regresión lineal múltiple. Materiales y métodos. El estudio se desarrolló en Llano Brenes, Alajuela, Costa Rica. Se instalaron sensores de reflectometría de dominio temporal (TDR) y registraron información cada veinte minutos de humedad de suelo desde noviembre 2018 a diciembre 2019. El suelo se clasificó a nivel taxonómico como Lithic Ustorthents, en una finca con cultivo de café en producción. Se tomaron muestras de suelo no disturbadas para la calibración de los TDR y se realizó un análisis de estabilidad temporal. El primer modelo (RLM1) fue una regresión lineal múltiple con variables meteorológicas, en el segundo modelo (RLM2) además de las variables meteorológicas, se separó la precipitación en subperíodos, los cuales se introdujeron como variables “dummy”, mientras que el tercer modelo (PCA) consistió en un análisis de componentes principales y un modelo de regresión lineal. Resultados. Los modelos RLM2 (R2 = 0,838) y PCA (R2 = 0,823) presentaron un mejor desempeño en comparación con el modelo RLM1 (R2 = 0,540). Sin embargo, el modelo RLM2 se consideró más útil, debido a su simplicidad y a que presentó los mejores indicadores de bondad de ajuste. Conclusión. Los modelos de regresión lineal con variables meteorológicas permitieron estimar la humedad del suelo, debido a que esta tendió a seguir los patrones estacionales y las variaciones de la precipitación, tal como se observó en el RLM2 con la separación de subperíodos.
Descargas
Citas
Amarasinghe, U. A., Hoanh, C. T., D’haeze, D., & Hung, T. Q. (2015). Toward sustainable coffee production in Vietnam: More coffee with less water. Agricultural Systems, 136, 96−105. https://doi.org/10.1016/j.agsy.2015.02.008
Antonucci, F., Pallottino, F., Costa, C., Rimatori, V., Giorgi, S., Papetti, P., & Menesatti, P. (2011). Development of a rapid soil water content detection technique using active infrared thermal methods for in-field applications. Sensors, 11(11), 10114−10128. https://doi.org/10.3390/s111110114
Bishara, A. J., & Hittner, J. B. (2017). Confidence intervals for correlations when data are not normal. Behavior Research Methods, 49, 294−309. https://doi.org/10.3758/s13428-016-0702-8
Brocca, L., Ciabatta, L., Massari, C., Camici, S., & Tarpanelli, A. (2017). Soil moisture for hydrological applications: Open questions and new opportunities. Water, 9(2), Article 140. https://doi.org/10.3390/w9020140
Brocca, L., Melone, F., Moramarco, T., & Morbidelli, R. (2009). Soil moisture temporal stability over experimental areas in Central Italy. Geoderma, 148(3−4), 364−374. https://doi.org/10.1016/j.geoderma.2008.11.004
Brocca, L., Melone, F., Moramarco, T., & Morbidelli, R. (2010). Spatial-temporal variability of soil moisture and its estimation across scales. Water Resources Research, 46(2), Article 8016. https://doi.org/10.1029/2009WR008016
Campbell Sci. (2020). CS616 and CS625 water content reflectometers. Campbell Scientific, Inc.
Campbell Sci. Inc. (2016). CR800 Series Dataloggers. Campbell Scientific, Inc.
Chen, L., Messing, I., Zhang, S., Fu, B., & Ledin, S. (2003). Land use evaluation and scenario analysis towards sustainable planning on the Loess Plateau in China—Case study in a small catchment. CATENA, 54(1−2), 303−316. https://doi.org/10.1016/S0341-8162(03)00071-7
Chuenchooklin, S., Pangnakorn, U., & Soonthornnonda, P. (2019). Comparative study using the 2-hydrological models with the global weather in a small watershed, a case study in the upper Tha Chin River Basin, Thailand. World Journal of Engineering and Technology, 7(2), 21−26. https://doi.org/10.4236/wjet.2019.72B003
Crow, W. T., & Yilmaz, M. T. (2014). The Auto-Tuned Land Data Assimilation System (ATLAS). Water Resources Research, 50(1), 371−385. https://doi.org/10.1002/2013WR014550
de Queiroz, M. G., Freire da Silva, T. G., Zolnier, S., da Rosa Ferraz Jardim, A. M., de Alves Souza, C. A., do Nascimento Araújo Júnior, G., Florentino de Morais, J. E., & Bastos de Souza, L. S. (2020). Spatial and temporal dynamics of soil moisture for surfaces with a change in land use in the semi-arid region of Brazil. CATENA, 188, Article 104457. https://doi.org/10.1016/j.catena.2020.104457
Devore, J. L., & Berk, K. N. (2012). Modern mathematical statistics with applications. Springer. https://doi.org/10.1007/978-1-4614-0391-3
Dong, Y., Miller, S., & Kelley, L. (2020). Performance evaluation of soil moisture sensors in coarse- and fine-textured Michigan agricultural soils. Agriculture, 10(12), Article 598. https://doi.org/10.3390/agriculture10120598
Forkuor, G., Hounkpatin, O. K. L., Welp, G., & Thiel, M. (2017). High resolution mapping of soil properties using remote sensing variables in South-Western Burkina Faso: A comparison of machine learning and multiple linear regression models. PLoS ONE, 12(1), Article e0170478. https://doi.org/10.1371/journal.pone.0170478
García, G., Brogioni, M., Venturini, V., Rodríguez, L., Walker, E., & Graciani, S. (2016). Determinación de la humedad de suelo mediante regresión lineal múltiple con datos TerraSAR-X. Revista de la Asociación Española de Teledetección, 46, 73−81. http:/doi.org/10.4995/raet.2016.4024
Heathman, G. C., Cosh, M. H., Han, E., Jackson, T. J., McKee, L., & McAfee, S. (2012). Field scale spatiotemporal analysis of surface soil moisture for evaluating point-scale in situ networks. Geoderma, 170, 195−205. https://doi.org/10.1016/j.geoderma.2011.11.004
Heathman, G. C., Starks, P. J., Ahuja, L. R., & Jackson, T. J. (2003). Assimilation of surface soil moisture to estimate profile soil water content. Journal of Hydrology, 279(1−4), 1−17. https://doi.org/10.1016/S0022-1694(03)00088-X
Huang, X., Shi, Z. H., Zhu, H. D., Zhang, H. Y., Ai, L., & Yin, W. (2016). Soil moisture dynamics within soil profiles and associated environmental controls. CATENA, 136, 189−196. https://doi.org/10.1016/j.catena.2015.01.014
Instituto Meteorológico Nacional. (2017). Estudio de cuencas hidrográficas de Costa Rica. Cuenca río Jesús María. http://cglobal.imn.ac.cr/documentos/publicaciones/EstudioCuencas/EstudioCuencas-cuencaRioJesusMaria.pdf
Kargas, G., & Soulis, K. X. (2012). Performance analysis and calibration of a new low-cost capacitance soil moisture sensor. Journal of Irrigation and Drainage Engineering, 138(7), 632−641. https://doi.org/10.1061/(ASCE)IR.1943-4774.0000449
Khan, M. A., Kakar, E., Baloch, D. M., & Azad, S. U. D. (2011). Calibration of Time Domain Reflectometry (TDR) soil moisture point probe for two soils. Journal of Applied and Emerging Sciences, 2(1), 19−26.
Legates, D. R., & McCabe, G. J. (1999). Evaluating the use of “goodness-of-fit” Measures in hydrologic and hydroclimatic model validation. Water Resources Research, 35(1), 233−241. https://doi.org/10.1029/1998WR900018
Martínez-Fernández, J., & Ceballos, A. (2005). Mean soil moisture estimation using temporal stability analysis. Journal of Hydrology, 312(1−4), 28−38. https://doi.org/10.1016/j.jhydrol.2005.02.007
Masarirambi, M. T., Chingwara, V., & Shongwe, V. D. (2009). The effect of irrigation on synchronization of coffee (Coffea arabica L.) flowering and berry ripening at Chipinge, Zimbabwe. Physics and Chemistry of the Earth, Parts A/B/C, 34(13), 786−789. https://doi.org/10.1016/j.pce.2009.06.013
McKenzie, N., & Austin, M. P. (1993). A quantitative Australian approach to medium and small scale surveys based on soil stratigraphy and environmental correlation. Geoderma, 57(4), 329−355. https://doi.org/10.1016/0016-7061(93)90049-Q
Mckenzie, N. J., & Macleod, D. A. (1989). Relationships between soil morphology and soil properties relevant to irrigated and dryland agriculture. Soil Research, 27(2), 235−258. https://doi.org/10.1071/sr9890235
McKenzie, N. J., & Ryan, P. J. (1999). Spatial prediction of soil properties using environmental correlation. Geoderma, 89(1), 67−94. https://doi.org/10.1016/S0016-7061(98)00137-2
McKenzie, N., Smettem, J., & Ringrose-Voase, A. J. (1991). Evaluation of methods for inferring air and water properties of soils from field morphology. Australian Journal of Soil Research, 29(5), 587−602. https://doi.org/10.1071/SR9910587
Mojid, M. A., & Cho, H. (2002). Response of the core and shield rods of time-domain reflectometry probe to transverse soil-water content heterogeneity. Journal of Hydrology, 262(1−4), 21−27. https://doi.org/10.1016/S0022-1694(01)00604-7
Molina-Campos, E. (2015). Determinación y cuantificación de la tasa de erosión laminar en cafetales ubicados en la cuenca del Río Jesús María [Tesis de Grado, no publicada]. Universidad de Costa Rica.
Montoani Silva, B., Oliveira, G. C., Serafim, M. E., Silva, É. A., Gontijo Guimarães, P. T., Batista Melo, L. B., Darrell Norton, L., & Curi, N. (2019). Soil moisture associated with least limiting water range, leaf water potential, initial growth and yield of coffee as affected by soil management system. Soil and Tillage Research, 189, 36−43. https://doi.org/10.1016/j.still.2018.12.016
Naranjo, M. E., & Ataroff, M. (2015). Calibración de equipos TDR para su uso en suelos no disturbados. Interciencia, 40(6), 416−422.
Nash, J., & Sutcliffe, J. (1970). River flow forecasting through conceptual models part I — A discussion of principles. Journal of Hydrology, 10(3), 282−290. https://doi.org/10.1016/0022-1694(70)90255-6
Parker, R. I. (2006). Increased reliability for single-case research results: Is the bootstrap the answer? Behavior Therapy, 37(4), 326−338. https://doi.org/10.1016/j.beth.2006.01.007
Qiu, Y., Fu, B., Wang, J., & Chen, L. (2001). Spatial variability of soil moisture content and its relation to environmental indices in a semi-arid gully catchment of the Loess Plateau, China. Journal of Arid Environments, 49(4), 723−750. https://doi.org/10.1006/jare.2001.0828
Qiu, Y., Fu, B., Wang, J., & Chen, L. (2003). Spatiotemporal prediction of soil moisture content using multiple-linear regression in a small catchment of the Loess Plateau, China. CATENA, 54(1-2), 173−195. https://doi.org/10.1016/S0341-8162(03)00064-X
Qiu, Y., Fu, B., Wang, J., Chen, L., Meng, Q., & Zhang, Y. (2010). Spatial prediction of soil moisture content using multiple-linear regressions in a gully catchment of the Loess Plateau, China. Journal of Arid Environments, 73(2), 208−220. https://doi.org/10.1016/j.jaridenv.2009.08.003
R Core Team. (2020). R: A language and environment for statistical computing. R Foundation for Statistical Computing. https://www.R-project.org/
Robinson, D. A., Jones, S. B., Wraith, J. M., Or, D., & Friedman, S. P. (2003). A Review of advances in dielectric and electrical conductivity measurement in soils using Time Domain Reflectometry. Vadose Zone Journal, 2(4), 444−475. https://doi.org/10.2136/vzj2003.4440
Russell, C. J., & Dean, M. A. (2000). To log or not to log: bootstrap as an alternative to the parametric estimation of moderation effects in the presence of skewed dependent variables. Organizational Research Methods, 3(2), 166−185. https://doi.org/10.1177%2F109442810032002
Sacardi Biudes, M., Vourlitis, G. L., Gomes Machado, N., Zanella de Arruda, P. H., Rodrigues Neves, G. A., de Almeida Lobo, F., Usher Neale, C. M., & de Souza Nogueira, J. (2015). Patterns of energy exchange for tropical ecosystems across a climate gradient in Mato Grosso, Brazil. Agricultural and Forest Meteorology, 202, 112−124. https://doi.org/10.1016/j.agrformet.2014.12.008
Soil Survey Staff. (2014). Keys to soil taxonomy. United States Department of Agriculture.
Sun, F., Lü, Y., Wang, J., Hu, J., & Fu, B. (2015). Soil moisture dynamics of typical ecosystems in response to precipitation: A monitoring-based analysis of hydrological service in the Qilian Mountains. CATENA, 129, 63−75. https://doi.org/10.1016/j.catena.2015.03.001
Tebbs, E., Gerard, F., Petrie, A., & De Witte, E. (2016). Emerging and potential future applications of satellite-based soil moisture products. In P. K. Srivastava, G. P. Petropoulos, & Y. H. Kerr (Eds.), Satellite Soil Moisture Retrieval (Chapter 19, pp. 379−400). Elsevier. https://doi.org/10.1016/B978-0-12-803388-3.00019-X
Udawatta, R. P., Anderson, S. H., Motavalli, P. P., & Garrett, H. E. (2011). Calibration of a water content reflectometer and soil water dynamics for an agroforestry practice. Agroforestry Systems, 82(1), 61−75. https://doi.org/10.1007/s10457-010-9362-3
Walker, J. P., Willgoose, G. R., & Kalma, J. D. (2004). In situ measurement of soil moisture: A comparison of techniques. Journal of Hydrology, 293(1−4), 85−99. https://doi.org/10.1016/j.jhydrol.2004.01.008
Waseem, M., Mani, N., Andiego, G., & Usman, M. (2017). A review of criteria of fit for hydrological models. International Research Journal of Engineering and Technology, 4(11), 1765−1772.
Webster, R., & McBratney, A. B. (1989). On the Akaike information criterion for choosing models for variograms of soil properties. Journal of Soil Science, 40(3), 493−496. https://doi.org/10.1111/j.1365-2389.1989.tb01291.x
Were, K., Bui, D. T., Dick, Ø. B., & Singh, B. R. (2015). A comparative assessment of support vector regression, artificial neural networks, and random forests for predicting and mapping soil organic carbon stocks across an Afromontane landscape. Ecological Indicators, 52, 394−403. https://doi.org/10.1016/j.ecolind.2014.12.028
Willmott, C. J. (1981). On the validation of models. Physical Geography, 2(2), 184−194. https://doi.org/10.1080/02723646.1981.10642213
Willmott, C. J., Ackleson, S. G., Davis, R. E., Feddema, J. J., Klink, K. M., Legates, D. R., O’Donnell, J., & Rowe, C. M. (1985). Statistics for the evaluation and comparison of models. Journal of Geophysical Research: Oceans, 90(C5), 8995−9005. https://doi.org/10.1029/JC090iC05p08995
Zaman, B., & McKee, M. (2014). Spatio-temporal prediction of root zone soil moisture using multivariate relevance vector machines. Open Journal of Modern Hydrology, 4(3), 80−90. https://doi.org/10.4236/ojmh.2014.43007
Zheng, W., Zhangzhong, L., Zhang, X., Wang, C., Zhang, S., Sun, S., & Niu, H. (2017, August 12-15). A review on the soil moisture prediction model and its application in the information system [Conferencia internacional]. International Conference on Computer and Computing Technologies in Agriculture, Jilin, China. https://doi.org/10.1007/978-3-030-06137-1_32
Zucco, G., Brocca, L., Moramarco, T., & Morbidelli, R. (2014). Influence of land use on soil moisture spatial–temporal variability and monitoring. Journal of Hydrology, 516, 193−199. https://doi.org/10.1016/j.jhydrol.2014.01.04
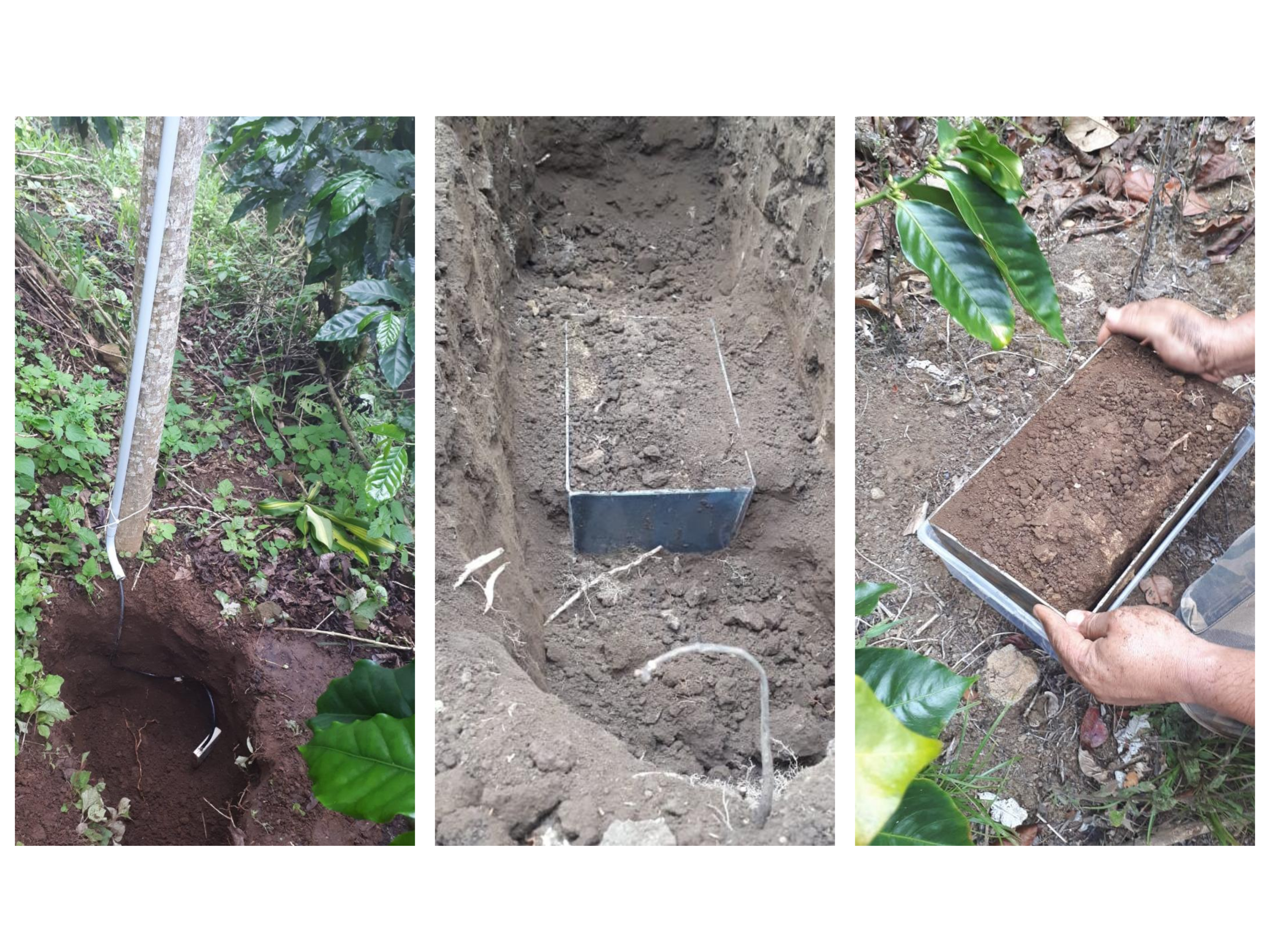
Archivos adicionales
Publicado
Cómo citar
Número
Sección
Licencia
1. Política propuesta para revistas de acceso abierto
Los autores/as que publiquen en esta revista aceptan las siguientes condiciones:
- Los autores/as conservan los derechos morales de autor y ceden a la revista el derecho de la primera publicación, con el trabajo registrado con la licencia de atribución, no comercial y sin obra derivada de Creative Commons, que permite a terceros utilizar lo publicado siempre que mencionen la autoría del trabajo y a la primera publicación en esta revista, no se puede hacer uso de la obra con propósitos comerciales y no se puede utilizar las publicaciones para remezclar, transformar o crear otra obra.
- Los autores/as pueden realizar otros acuerdos contractuales independientes y adicionales para la distribución no exclusiva de la versión del artículo publicado en esta revista (p. ej., incluirlo en un repositorio institucional o publicarlo en un libro) siempre que indiquen claramente que el trabajo se publicó por primera vez en esta revista.
- Se permite y recomienda a los autores/as a publicar su trabajo en Internet (por ejemplo en páginas institucionales o personales) antes y durante el proceso de revisión y publicación, ya que puede conducir a intercambios productivos y a una mayor y más rápida difusión del trabajo publicado (vea The Effect of Open Access).